
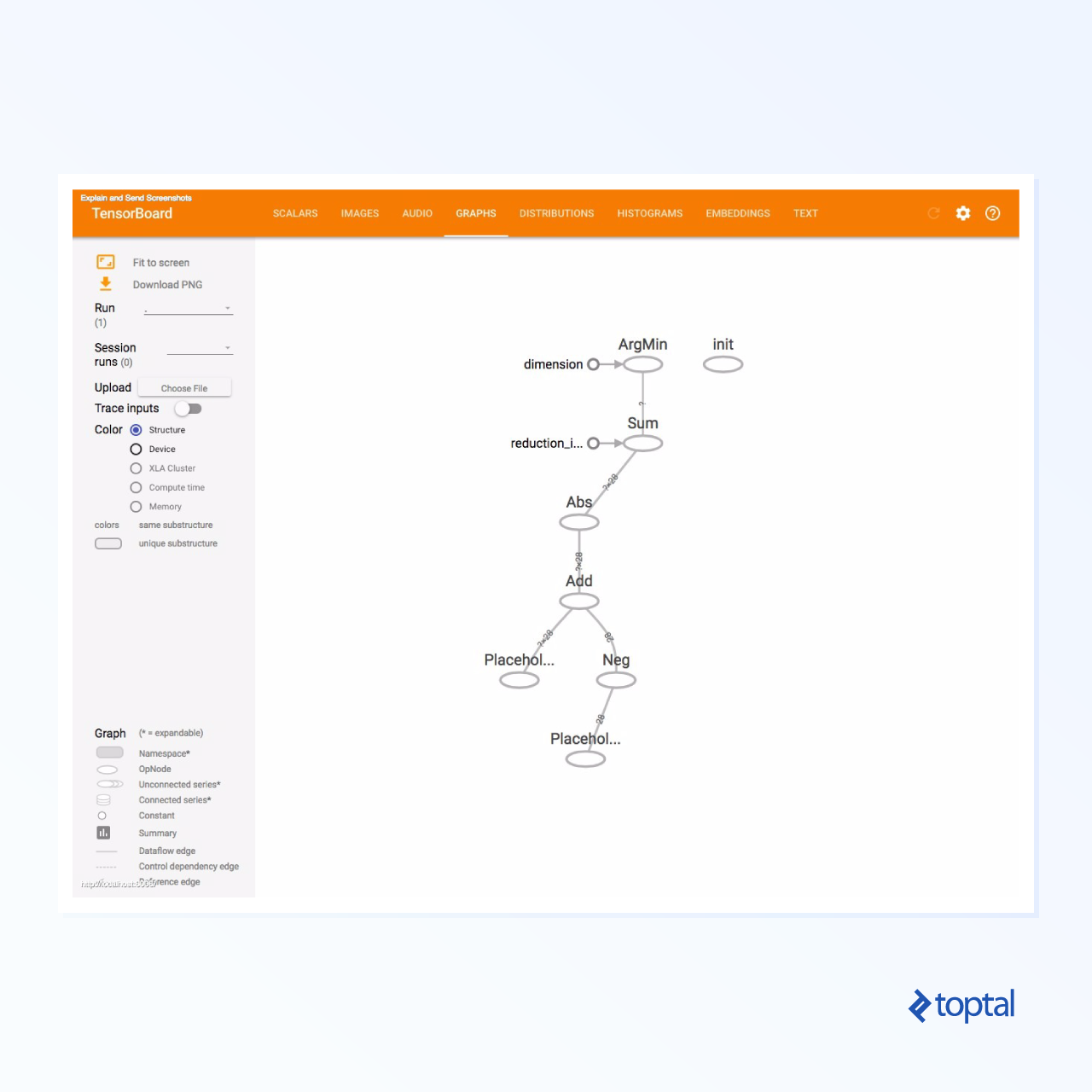
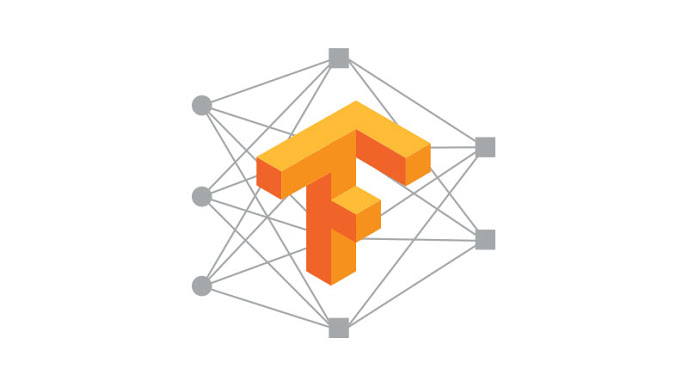
Image Augmentation with ImageDataGenerator Let’s look at a couple of ways to put image augmentation to work, and then apply it to the Arctic-wildlife model presented in the previous post. Keras has built-in support for data augmentation with images. You can see why presenting the same image to a model in different ways might make the model more adept at recognizing hot dogs, regardless of how the hot dog is framed. The figure below shows the effect of applying random transforms to a hot-dog image. This can increase a model’s ability to generalize with little to no impact on training time. Images are transformed differently in each epoch, so if you train for 10 epochs, the network sees 10 different variations of each training image. Keras makes it easy to randomly transform training images provided to a network. It doesn’t always increase accuracy, but it frequently does.
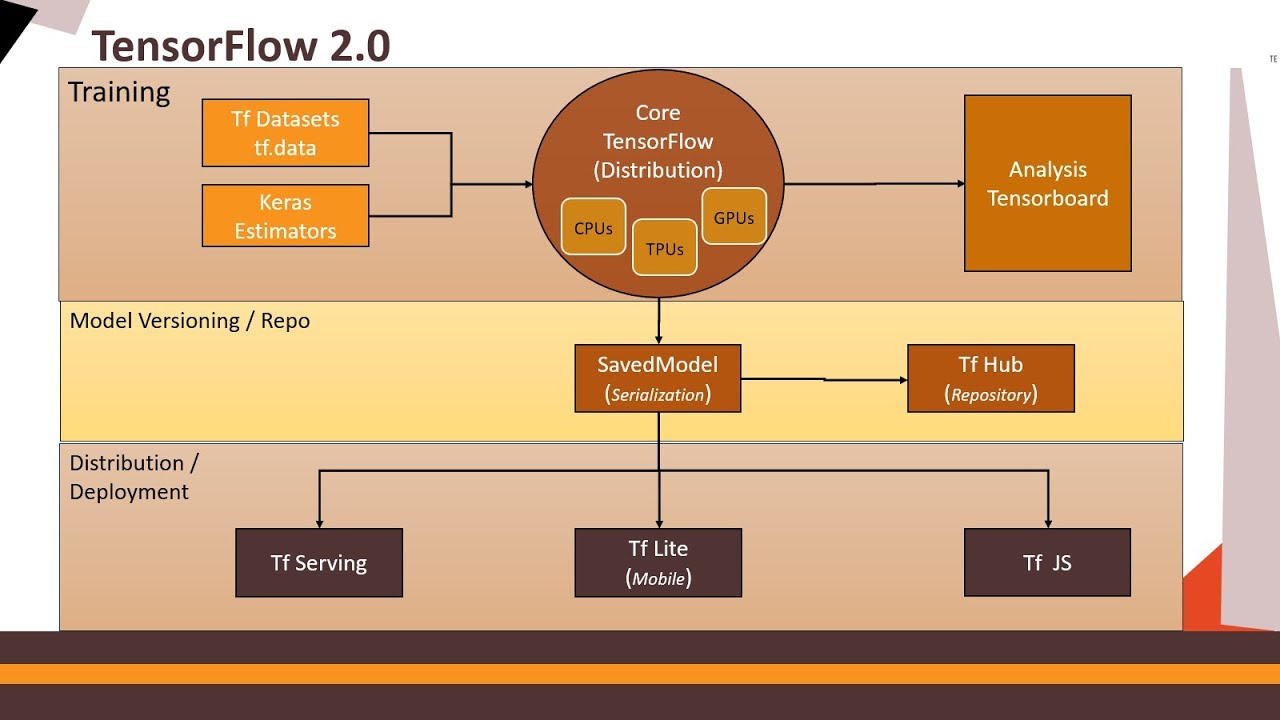
Rather than scare up more training images, you can rotate, translate, and scale the images you have. But if the training images don’t include photos with the bear’s head aligned differently or tilted at different angles, the model might have difficulty classifying the photo. A model might be able to recognize a polar bear if the bear’s head is perfectly aligned in center of the photo. With just 100 or samples of each class, there isn’t a lot of diversity among images. This feature, however, can also be a bug. One of the benefits of transfer learning is that it can do a lot with relatively few images.
Tensorflow image data generator how to#
My previous post demonstrated how to use transfer learning to build a model that with just 300 training images can classify photos of three different types of Arctic wildlife with 95% accuracy.
